Harnessing AI and automation in refining and applying location data
How OS is using AI to provide quality geospatial data and meet its users' needs
To keep pace with our users’ demands, we need to design and execute workflows for greater effectiveness, while safeguarding the reliability and trust in the data. Better data, and more destinations for our data, is core to our strategy.
We have therefore considered our value chain and our key services, and broken them down into five key areas: positioning, sourcing, refining, distributing, and applying.
This presents clear opportunities for us to leverage the power of AI and automation in refining and applying data.
Computer Vision and Machine Learning
We use two distinct methodologies of AI to extract required features from images: Computer Vision (CV) and Machine Learning (ML). When using Computer Vision methods, we develop repeatable rules or algorithms to process the images through programmed code. This method works well when we have a good understanding about the relationship between the input images and the output features. When this relationship is less clear, we enlist the help of Machine Learning to provide the answer. There are many ways to train a ML model, but a common approach involves providing examples of input and the related desired labelled output. Once trained, the model can be used to detect the output in new images without the coded rules necessary for CV methods.
We are one of the very few organisations that has already deployed CV and ML techniques. Our method can explore the possibility of performing the heavy lifting of feature extraction, on images to cover a national scale, that would otherwise take a large group of trained professionals.
This year is the first time we've productionised the workflow to deliver a national dataset to the market (OS Land Cover); and the first time we've used ML in that workflow. More of this will happen going forward, to drive currency and more accurate data, and variation in datasets. Better data, to solve the right problems, and new data types to enable more destinations.
Once we have achieved the desired output from the trained model, our efforts shift to tracking the performance over time to ensure we maintain quality levels despite imperceptible differences in the input images. This type of performance monitoring is an emerging field in ML and will therefore require us to adapt as we understand more.
Like everyone else, we're grappling with the massive change in accessibility to Generative AI capability that has been presented in the last 18 months. Large Language Models have enabled more natural ways to interact with technology, including how some of our users find and query geospatial data.
While this democratisation is positive, it opens the door to greater competition, so it’s important for us to have a closer link between the Engineering, Research, and User Experience teams to ensure we are leading the way with what is possible.
Our commitment to providing quality and trusted foundational data will continue to serve as a valuable resource for decision-makers everywhere.
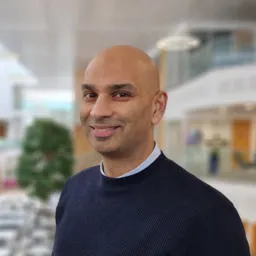
Chief Technology Officer
Further support
For further help about how our data can transform your organisation, contact us today